The Science Behind AI Image Generators
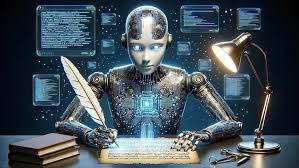
Artificial Intelligence (AI) has revolutionized how we interact with technology, and image generation is one of its most fascinating applications. But how do AI image generators work, and what makes them so effective? Let’s explore the science behind these cutting-edge tools.
Understanding AI Image Generators
At their core, AI image generators rely on neural networks, particularly a type called Generative Adversarial Networks (GANs). Introduced by Ian Goodfellow in 2014, GANs consist of two components:
- The Generator: Creates images from random noise.
- The Discriminator: Evaluates these images and determines whether they’re real or artificially generated.
These two components work in tandem, improving each other’s performance. The generator aims to create images so realistic that the discriminator cannot tell they’re fake. Over time, this adversarial process refines the generated images to an impressive degree of realism.
AI models like DALL-E, Stable Diffusion, and MidJourney have pushed these capabilities further. They combine GANs with natural language processing (NLP) to generate images based on text descriptions. For instance, typing “a serene mountain landscape at sunset” into a free AI image generator can yield stunningly accurate visuals.
The Role of Training Data
AI models learn by analyzing vast amounts of data. For image generators, this means training on millions of images paired with corresponding labels or descriptions. This extensive dataset allows the AI to recognize patterns, understand textures, and replicate artistic styles.
However, the quality and diversity of the training data significantly influence the output. Models trained on diverse datasets can create more versatile and culturally nuanced images, while limited datasets may result in biased or unrealistic visuals.
Real-World Applications
AI image generators are more than just a novelty; they have practical applications across various industries:
- Marketing and Advertising: Brands use AI-generated visuals to create personalized and engaging campaigns.
- Entertainment: Game developers and filmmakers leverage these tools to design unique characters and immersive environments.
- Education: AI visuals enhance e-learning by providing custom illustrations for complex concepts.
Ethical Considerations
While AI image generation is groundbreaking, it’s not without challenges. Concerns about copyright infringement, misuse for deepfakes, and potential biases in generated content highlight the need for ethical guidelines and transparent development practices.
What’s Next for AI Image Generators?
The future of AI image generation is promising. Researchers are working on improving model efficiency, reducing computational requirements, and enabling higher-quality outputs. As these tools become more accessible, they will likely transform creative industries and everyday workflows.
Final Thoughts
AI image generators are a testament to how far technology has come in replicating human creativity. By understanding the science behind them, we can better appreciate their capabilities and navigate their challenges responsibly. Whether you’re a marketer, artist, or tech enthusiast, these tools open up new horizons for innovation.